Did you know that it’s possible to perform a 3D reconstruction of an entire city using Computer Vision techniques from photos found online? I mean, photos that other people have posted on social media platforms such as Flickr, Instagram, among others.
Experimental results published in the paper “Building Rome in a Day” revealed that it’s possible to reconstruct entire cities, with up to 150,000 images, in less than a day, using a computing cluster with 500 cores. If you want to learn more about this Computer Vision technique, follow my analysis in this post!
3D City Reconstruction with Computer Vision
In 2009, at the International Conference on Computer Vision hosted by IEEE (Institute of Electrical and Electronics Engineers), the article “Building Rome in a Day” was published. In it, an innovative method was introduced that uses computer vision to create high-resolution 3D models of entire cities from 2D images sourced online.
The authors showcased the system’s effectiveness across various cities, including Rome, hence the paper’s title. Using a vast amount of online-sourced images, the proposed method was able to automatically reconstruct detailed 3D models of the cities.
Compared to other 3D modeling methods, the authors’ proposed method has numerous advantages. Firstly, it’s fully automated and can efficiently handle large datasets of high-resolution images, which means it can be used to model entire cities with relatively little human effort. Furthermore, the automated photogrammetry approach is less intrusive than other 3D data collection techniques, such as laser scanning, which can be crucial in sensitive or historical areas.
The system employs a series of parallel and distributed matching and reconstruction algorithms, designed to maximize parallelism at every step of the process and minimize serialization bottlenecks. This approach allows for efficient scalability both in terms of the problem size and available computing resources.
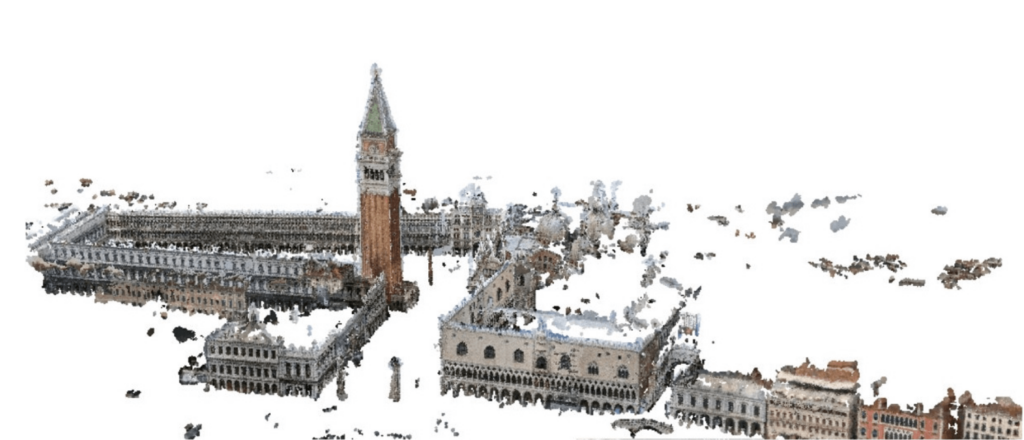
City-scale 3D reconstruction has been previously explored in computer vision literature and is widely used in platforms such as Google Earth and Microsoft’s Virtual Earth. However, existing large-scale Structure from Motion (SfM) systems operate with data from structured sources, like aerial photos taken by survey aircraft or images captured by moving vehicles. These systems rely on photos taken with calibrated cameras and often utilize other sensors, such as GPS and inertial navigation units, significantly simplifying the involved calculations.
On the other hand, images sourced from the web don’t have these simplifying features. They are captured with various cameras, under fluctuating lighting conditions, and often lack geographical or camera calibration information. This variability makes photo collections harder to work with for SfM purposes but also makes them an extremely rich source of information about the world. These images capture noteworthy aspects worth photographing, including interiors and artifacts, in addition to exteriors.
Given these characteristics, the paper represents a significant advancement in constructing 3D city models and has important implications in certain areas like cartography, virtual tourism, and urban planning.
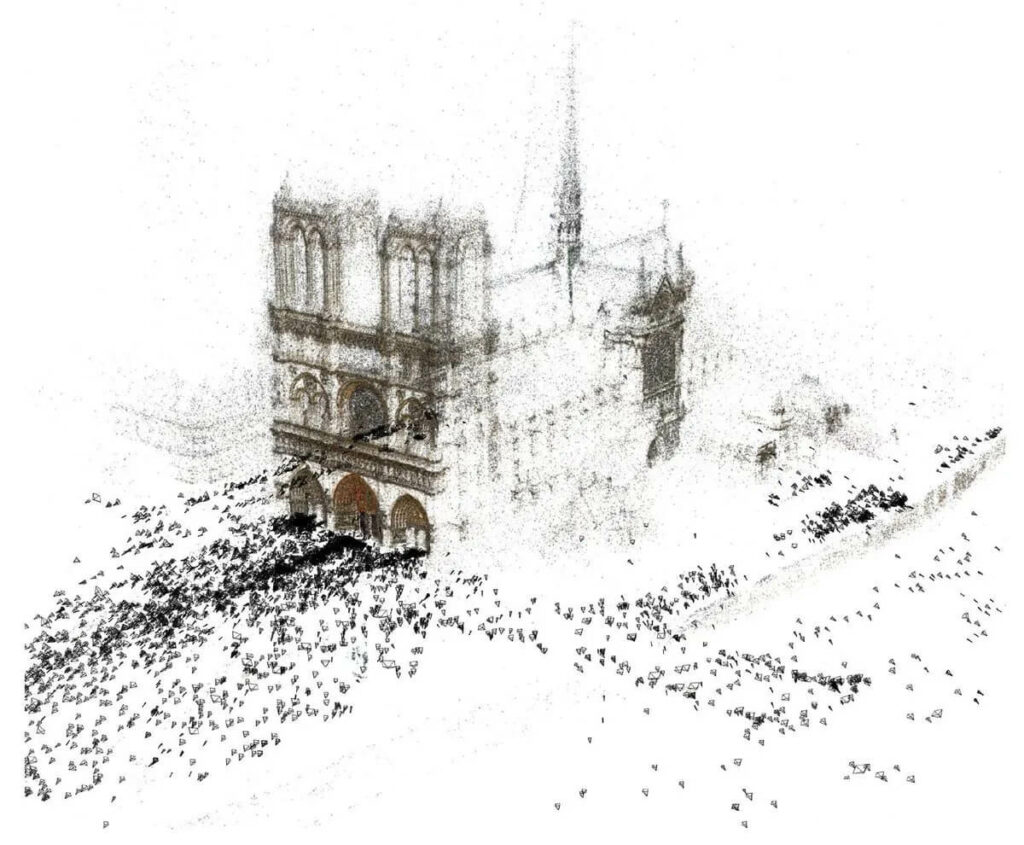
Understanding the Methodology for 3D City Reconstruction
There are three stages in 3D city reconstruction, in order: image capture, image matching, and 3D reconstruction. The process begins by capturing a large number of images of the desired city to model. In this case, the authors tested their method on the city of Rome and utilized over 150,000 images from Flickr.
These images are then loaded into software that uses feature matching techniques (image matching algorithms) to find common points in each image and build a network of points of interest. These points are used to calculate the camera’s position and orientation in each image and to create an approximate 3D point cloud of the scene.
Next, the software uses the images and the approximate point cloud to generate a dense 3D mesh of the scene, representing the city’s surface with precise details. To do this, the method proposed by the authors uses a technique called “stereo matching”, which finds matches between images using epipolar geometry and luminance differences between images.
Finally, the authors apply a texturing technique to add color and texture details to the 3D mesh. This is done automatically, using the original images to extract color and texture information and project them onto the 3D mesh. The end result is a highly detailed and realistic 3D model of the city, which can be used for visualization, urban planning, natural disaster simulations, among other purposes.
The process of 3D city reconstruction from images can be divided into several steps, as follows:
- Image capture: the first step is to capture images of the city from different angles and positions using handheld cameras or cars equipped with cameras. It is important to capture enough images to cover the entire area of the city you want to reconstruct.
- Camera calibration: before processing the images, it is necessary to calibrate the camera to determine its intrinsic and extrinsic parameters. Intrinsic parameters include the camera’s focal length and principal point, while extrinsic parameters include the camera’s position and orientation relative to the scene. Calibration can be done using calibration standards or self-calibration techniques.
- Feature matching: then, the images are processed to find matches between points of interest in image pairs. This can be done using feature matching techniques such as SIFT (Scale-Invariant Feature Transform) or SURF (Speeded Up Robust Features), which identify points of interest in each image and find matches between them in image pairs.
- Triangulation: after finding the matches, the corresponding 3D points can be estimated using triangulation. Triangulation is a method to find the 3D position of a point from its projection in two or more images. These 3D points are usually imprecise and noisy but form an approximate point cloud of the scene.
- Point cloud optimization: the estimated point cloud can be refined using optimization techniques such as energy minimization or graph optimization to improve the 3D position accuracy of each point.
- 3D mesh generation: after obtaining a refined 3D point cloud, it is possible to generate a 3D city mesh using triangulation or Delaunay techniques, which connect neighboring 3D points to form a continuous 3D surface.
- 3D mesh texturing: finally, the 3D mesh can be textured using the input images to create a visually realistic representation of the reconstructed city.
Various Applications of 3D Reconstruction
3D city reconstruction has revolutionized various areas of work, opening doors to new possibilities and practical applications. From urban planning to the tourism industry and archaeology, this technology has provided significant advancements and innovative solutions. In this section, we will discuss some of the main applications of 3D city reconstruction and how they are transforming various sectors.
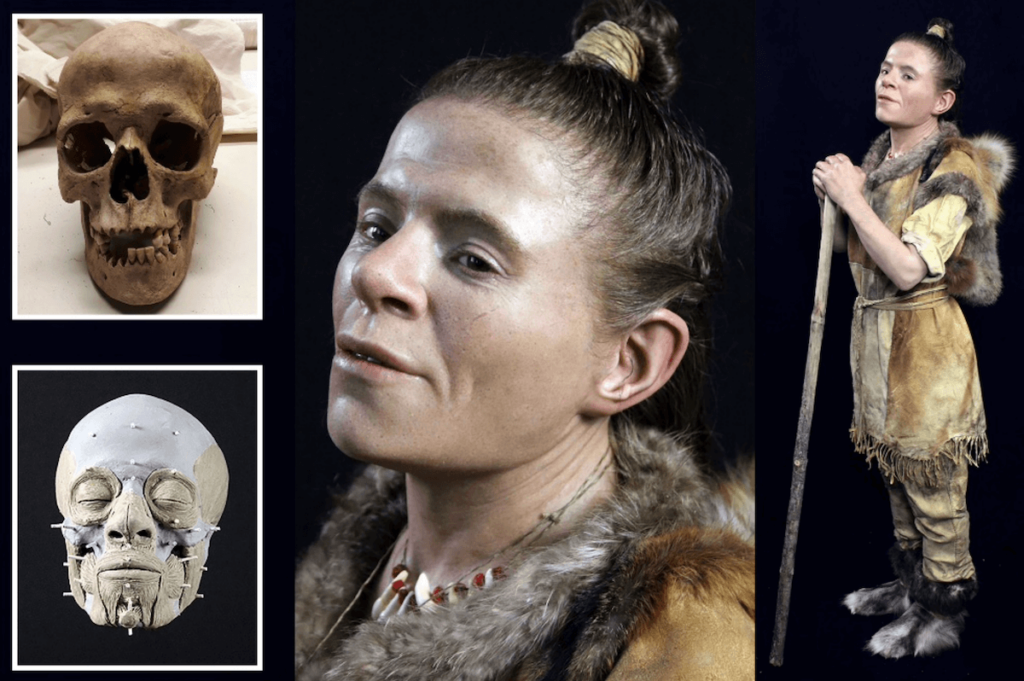
3D city reconstruction technology has been widely used in various sectors, as illustrated in the list below.
- Urban planning and architecture: 3D reconstructions can assist architects and urban planners in designing constructions according to area characteristics and existing structures. For instance, municipal authorities can use this technology to visualize how new constructions will affect the city’s look and traffic, as well as to identify flood or natural disaster risk areas.
- Geospatial data analysis: three-dimensional models can be integrated with other data sources, such as maps and satellite data, to provide insights into traffic, land use, and population density.
- Archaeology and cultural heritage: 3D city reconstruction can be used for historical and cultural preservation purposes, making it possible to create accurate three-dimensional models of ancient buildings or archaeological sites. This allows them to be studied and virtually preserved, even if they have been demolished or damaged.
- Simulation and modeling: 3D models can be used to simulate and model urban scenarios and help researchers understand how cities evolve over time.
- Machine learning and computer vision: 3D models can be used to train machine learning and computer vision algorithms to recognize objects and patterns in urban environments.
- Tourism: this technology can be used in the tourism industry to create immersive and educational experiences. For example, a museum can create a virtual tour of a historic city, allowing visitors to see what the city looked like in the past. Similarly, tourism agencies can create interactive tour guides that assist visitors in exploring the city and its attractions.
- Games: 3D city reconstruction is often used in the gaming industry to create realistic environments. For instance, a game might use a 3D reconstruction of an existing city as the basis for creating a virtual world for players to explore. Similarly, a historical game might use a 3D reconstruction of an ancient city to create an authentic environment.
- Security: Used in emergency planning and disaster response, allowing rescue teams to view affected sites and plan rescue operations more efficiently. Three-dimensional models can be used to predict how disasters would affect different city areas and what the safest escape routes would be. Likewise, it can enhance public safety, allowing authorities to monitor and analyze traffic patterns, pedestrian flow, and criminal activities in urban areas.
- Public Budget: Planning the use of public resources, such as public lighting, traffic monitoring, and waste management.
In short, 3D city reconstruction technology has a profound impact on various areas, enabling more detailed analyses, efficient planning, and immersive experiences. As technology advances and becomes more accessible, the use of 3D city reconstruction is expected to further expand, covering new sectors and applications. Without a doubt, 3D city reconstruction is reshaping the world around us, creating new opportunities and paving the way for future innovations.
Challenges and Limitations
Obviously, every technology has its limitations and challenges to overcome. The limitations of technology used in 3D city reconstruction are closely linked to the images, both numerically and qualitatively.
To model an entire city in detail, it’s necessary to capture a large number of images from different angles and positions, which can be a logistical and cost challenge. There is also a dependency on high-quality photos (which is logistically and economically challenging in remote areas), taken under good lighting and weather conditions to achieve good results. Moreover, the quality of the final model can be affected by camera calibration, which, if incorrect, can lead to errors in determining the camera’s position and orientation in each image, directly affecting the accuracy of the final modeling.
Privacy is a major concern when collecting aerial or ground images of urban areas. Because images may contain personal information, such as car license plates, faces, and even the internal layout of buildings, this could compromise citizens’ security and privacy. Therefore, it’s important for companies and institutions involved in creating 3D city reconstructions to establish clear guidelines for ethical data use and protect people’s privacy.
Perspectives and Opportunities
3D city reconstruction is a rapidly evolving area with a promising future, packed with opportunities for technological advances and new applications. Some of the main perspectives and opportunities are listed below.
- Increased Resolution and Accuracy: With advancements in imaging technology, cameras and sensors are expected to become more powerful, allowing for the collection of high-resolution and more accurate data. This will allow for even more detailed and accurate 3D reconstructions.
- Use of Drones and Robots: Using drones and robots for data collection can make the 3D reconstruction process more efficient and accurate, especially in hard-to-reach or dangerous areas.
- Machine Learning and Artificial Intelligence: Using machine learning and artificial intelligence techniques can help automate the 3D reconstruction process, allowing for the collection and processing of vast amounts of data more quickly and efficiently.
- Integration with Other Technologies: 3D city reconstruction can be integrated with other technologies, such as augmented and virtual reality, allowing users to interact with cities in an even more immersive and engaging manner.
- Applications in Emerging Areas: 3D city reconstruction has great potential for use in emerging areas like urban planning, environmental monitoring, natural disaster prevention, and risk assessment.
Conclusion
3D reconstruction and computer vision are becoming increasingly valuable tools in analyzing and preserving the past. As technology continues to progress, we are likely to see even more applications and innovations in this field, helping to unravel the mysteries of ancient civilizations and protect our historical heritage for future generations.
And for those who wish to delve deeper into 3D reconstruction, I definitely recommend reading my complete tutorial on ORB-SLAM, where I walk you through the step-by-step process of transforming images and videos into 3D maps.