On April 11, 1970, at 1:13 PM, NASA launched the Saturn V rocket from the John F. Kennedy Space Center, commencing the seventh manned mission of its Apollo Program – Apollo 13.
By that time, two other Apollo missions had already achieved lunar landings. Overall, the program included seven missions to the Moon, numbered Apollo 11 through Apollo 17.
However, a message transmitted to the Mission Control Center already indicated that something had changed.
- Jack Swigert: "Okay, Houston, we've had a problem here."
- CAPCOM (Jack R. Lousma): "This is Houston. Say again, please."
- Jim Lovell: "Ah, Houston, we've had a problem here. We've had a Main B Bus Undervolt."
The Apollo 13 astronauts did not know at the time, but oxygen tank number 2 had exploded. Besides being vital for the crew’s breathing, oxygen is used by the rocket’s propulsion systems.
NASA convened dozens of its top experts to devise a solution to this critical situation. The most immediate problem was to figure out a way to prevent carbon dioxide levels (or carbonic gas, CO2) from rising too quickly, which would certainly kill the three astronauts.
To make matters worse, these experts had to imagine a solution using only the equipment available on the spacecraft – such as plastic bags, paper boxes, and duct tape. The solution had to be so simple that the instructions given by the experts could be transmitted over the radio and replicated by the astronauts.
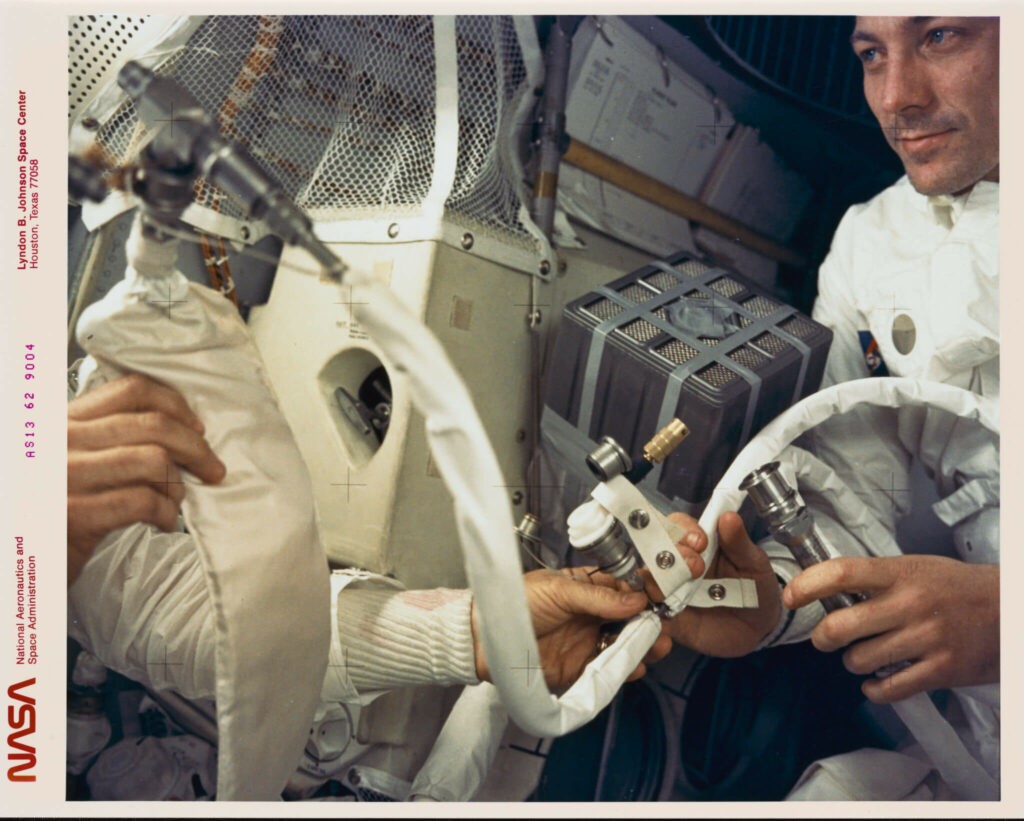
This dramatic moment was the stage for real clashes among the brightest engineers of this space agency. Every kind of solution presented was debated, considered, or discarded. Fortunately, thanks to the creativity of these specialists (ingenuity means, according to the Aulete Caldas digital dictionary: “the capacity to create, to invent. The skill of someone who is adept; dexterity”), all astronauts of the Apollo 13 mission managed to survive and return to Earth.
As one NASA scientist put it, ‘Apollo 13 demonstrated humanity’s capacity to solve complex problems with simple, ingenious solutions’. This aligns with the scientific principle of Occam’s Razor, which favors simpler explanations when multiple viable options exist.
Drawing Career Insights from the Apollo 13 Mission
This incident highlights a crucial lesson for both everyday and professional life: solutions, no matter how simple, are effective because, ultimately, they solve problems. Consider street running as an analogy: it doesn’t require expensive gear. Comfortable clothes and suitable sneakers, or even barefoot running depending on the terrain, suffice.
Similarly, the space engineers during Apollo 13 had to devise a solution using only the materials available on the spacecraft, after all, they wouldn’t have time (and it would be very expensive too) to bring materials from Earth to the Moon.
Believing you need every possible resource at your disposal can lead to procrastination and a slowdown in progress. In reality, you’ll never be 100% prepared with the best resources for every task. Scarcity and necessity are friends of optimization, efficiency – as that famous saying goes: “to get blood out of a stone”. Therefore, use what you have at the moment to build something.
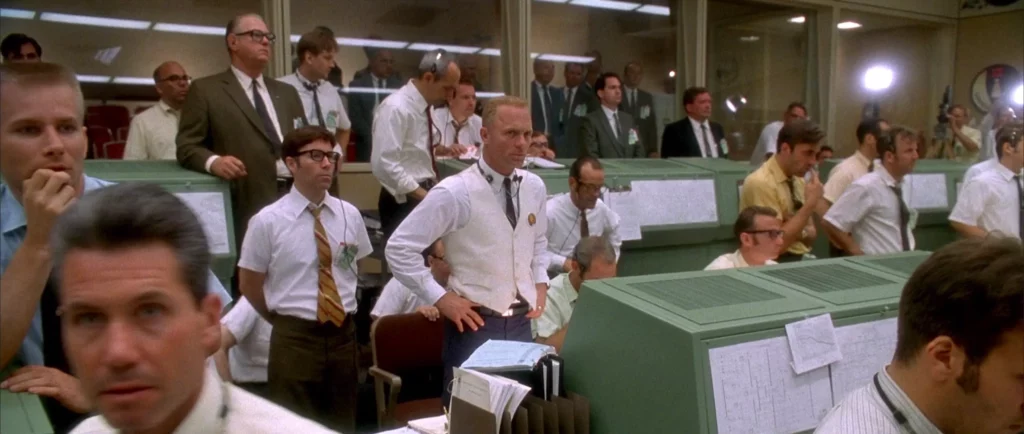
Applying this principle to your career, the key advice is to materialize your knowledge by producing tangible content. In a field as practical and evolving as machine learning, employers seek candidates who can demonstrate their knowledge through tangible projects, not just theoretical understanding.
Rethinking the Traditional Learning Model in Machine Learning
The insights I’m about to share are rooted in my personal experiences in the industry. I went through the same frustrations that you are experiencing at this point in your career, that’s why I created my first machine learning training programs. Numerous courses promise to transform learners into machine learning experts. Yet, I’ve consistently observed an overemphasis on theory, a lack of market readiness, and a scarcity of real-life applications.
“Apollo 13 demonstrated the human capacity to solve difficult problems with simple solutions”
A critical issue with many Data Science courses and materials is their focus solely on hard skills. That is, you learn to program, analyze data, plot graphs, build machine learning models, do deployments… and that’s it! Of course, knowing all this is very important. However, how to get hired? How to get a job?
Do you know what most people do? A common approach involves creating LinkedIn profiles, sending resumes indiscriminately, and continuously improving skills, yet often without receiving any response from employers. You might be wondering, ‘If this isn’t the effective approach, what is?’
Securing a Job in a Competitive Market: A Novel Approach
In today’s highly competitive job market, especially in the field of data science, traditional methods of job hunting are often insufficient. To truly stand out, one must adopt a proactive strategy that compels recruiters to take notice.This is where my ‘3P Methodology’ comes into play, an essential trio of Practice, Portfolio, and Personal Branding.
1) Emphasis on Practical Machine Learning Projects:
It is critical to focus on hands-on projects that utilize real-world data. While theoretical knowledge is foundational, it alone cannot fully prepare you for the demands of the industry. Engaging in practical projects not only enhances your skills but also demonstrates the real-world applicability and excitement of the field.
2) Your Portfolio Reflects Your Expertise
Reflect on the time you have dedicated to learning. How many of your projects have seen the light of day? In the AI arena, a GitHub portfolio is more than just a collection; it is a testament to your skills and commitment.
If your projects are not showcased, you are not fully leveraging your learning hours. In AI, your portfolio speaks louder than your resume. It should be a dynamic display of your abilities and dedication.
3) Building and Nurturing Your Personal Brand
Personal Branding is often overlooked, yet it is a key factor in establishing your authority and attracting attention from recruiters and headhunters. Actively engage in publishing content, making your expertise and insights known to the world.
Final Thoughts
Start publishing content immediately. Make people know who you are. Have an action plan to create a personal brand. I advocate the principle of Content Marketing for anyone wanting to differentiate themselves from all other professionals in the market.
To maximize your impact in the job market, start applying these principles from the outset. Focus on practical learning, diligently build and maintain your portfolio, and craft a personal brand that resonates with your professional aspirations. By doing so, you’ll not only stand out but also position yourself as a desirable candidate in the dynamic world of machine learning and AI.